Moving to Substack ➡️, Scaling Up 📈, Image Generation 🖼
Hi all,
Welp. Twitter plans to shut down Revue, my previous newsletter platform. I hope you like our new home, Substack. 🤗 I’m actually quite excited about the change. Substack allows comments on posts. We can now have actual discussions rather than just one-on-one email exchanges. Yay!
If you are already subscribed, everything should stay the same (I hope). If you are not, have a look around and see if you like things. All newsletter issues will stay free as before.
Here is some coverage of recent work on scaling up language models and text-based image generation that I did not get around to publishing (updated with some recent results).
Scaling up results 📈
As language models have become larger, they have outgrown some of the datasets we used to evaluate them. BIG-Bench, a two-year collaboration consisting of 204 tasks created by 442 authors aims to provide a diverse collection of tasks for the evaluation of current and future models.
Using 204 tasks for evaluation can be quite unwieldy, however, so recent work has already focused on 23 particularly challenging tasks where prior models did not outperform human annotators. It turns out that if you use chain-of-thought prompting—a recent method where you prompt a method to predict intermediate reasoning steps before producing the final answer—then recent large models surpass the average human annotator on up to 17/23 tasks. It seems we need to keep looking for more challenging evaluation tasks for large language models.
One thing that we have learned from training and evaluating large language models, is that "scale generally works". In other words, making models larger and training on more data usually leads to improved performance.
In some cases, this can lead to new behaviour. A small model may be bad at adding two numbers while a larger model may suddenly be able to perform the task with reasonable accuracy. In such cases, the linear performance curves that we would expect based on scaling laws are instead step-functions that transition from random to above-random performance at a certain model scale.
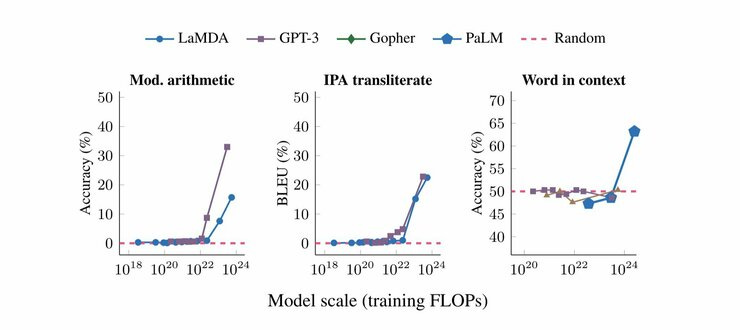
Such behaviour has been observed by recent large model papers such as LaMDA, GPT-3, Gopher, or PaLM. Wei et al. (2022) provide an overview of other types of emergent abilities that have been identified so far in current models.
Besides such emergent abilities, is there any other behaviour that may be surprising as we scale models up? The Inverse Scaling Prize, a recent competition awarded a prize of up to $100,000 if you find a task where performance goes down 📉 as models get larger.
A good way to narrow the search space is to look for tasks where an inductive bias is more important than more parameters. A minimal example for this is highlighted by @hardmaru. Given that the evaluation will focus on large language models, anything that cannot be learned from large amounts of text may be a good starting point. Nevertheless, large language models provide a good initialisation for various sequence modelling tasks such as RL (Reid et al., 2022) or can be easily learned together with other sequence tasks (Reed et al., 2022). So simply the fact that something is hard to learn from text alone does not mean that large LMs will not be able to learn it.
Scaling effects may also disappear or appear differently when investigated in a larger-scale setting. For some of the Inverse Scaling Prize winners, Wei et al. (2022) observe that the inverse scaling effect goes away when evaluated with 2x larger models and 5x more training compute. They also highlight the usefulness of chain-of-thought prompting to protect against inverse scaling. Inverse scaling thus depends on the specific training and test setting of a model.
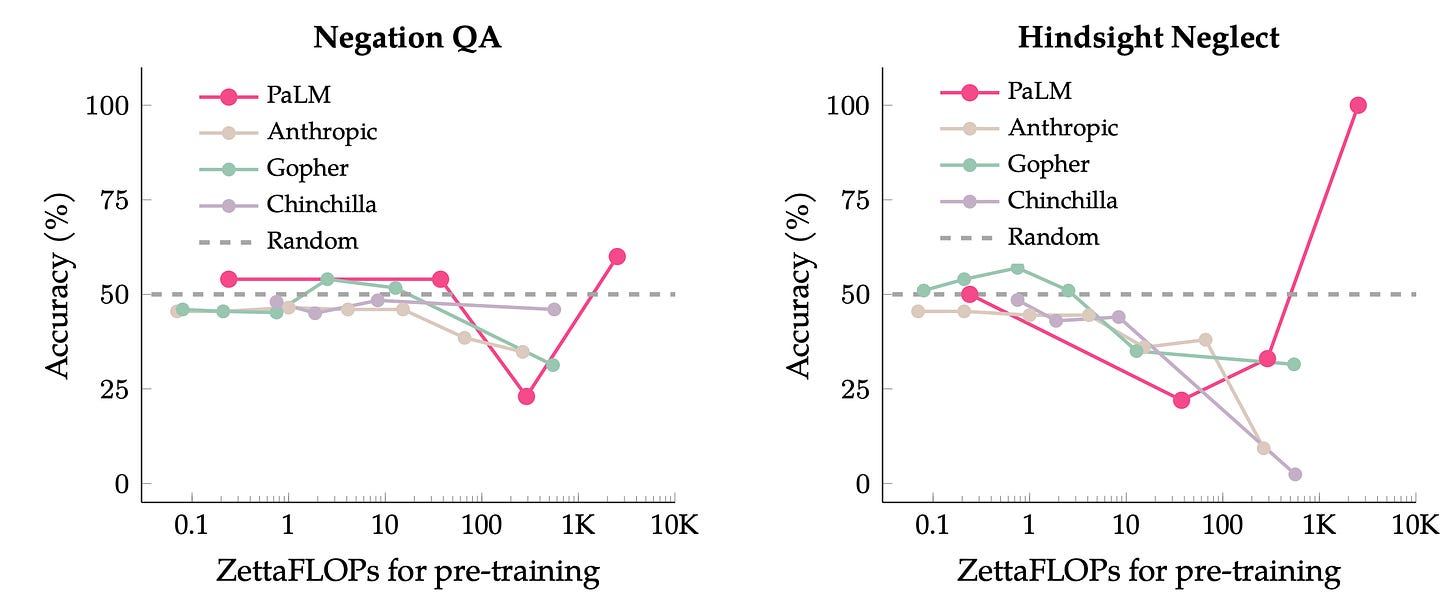
Image Generation is Heating Up 🔥
Imagen and Parti
After DALL-E 2, two new text-to-image models have been released by Google, Imagen (pronounced "imagine") and Parti (pronounced "par-tee"). Similar to DALL-E 2, Imagen is a diffusion model. Parti, on the other hand, is auto-regressive and more like a standard encoder-decoder language model (LM). It learns to decode images into visual tokens, which are then converted by a ViT-VQGAN model into an actual image.
As the blog post highlights, limitations of current text-to-image models include their inability to count reliably, to follow precise spatial instructions, and to deal with complex prompts.
Given the potential of these models, it is not difficult to envision strategies to shore up each of these weaknesses. These can include collecting data that explicitly represents each phenomenon, using distant supervision with large amounts of data, and more explicit representations of number or spatial attributes in current models.
Another thing that can be challenging with current models is to generate natural text. For instance, DALL-E often produces gibberish as can be seen below.
Daras et al. (2022) fed some of the gibberish text it produced back to DALL-E 2 as a prompt, discovering that the model indeed generates images related to vegetables. They argue that many such terms are consistent across prompts and thus DALL-E 2 has a "secret" vocabulary (a "secret language" in the original Twitter thread). In practice, such studies should be taken with a large grain of salt 🧂. Benjamin Hilton highlights that most terms are not consistent across different prompts. So while terms like "Apoploe vesrreaitais" may kind of look like a biological name for birds and thus result in mostly bird-related generations, most other terms produced by DALL-E 2 are just noise—and definitely do not have the properties of a language such as grammar, morphology, etc.
After all, we know that models learning to play referential games often come up with degenerate communication protocols consisting of arbitrary symbols (Chaabouni et al., 2022 is a nice recent study of the importance of scale for emergent communication). So I hope we go can turn down the hype and clickbaiting; let’s not go back to a time of newspapers reporting of AIs inventing a language that humans don't understand 🙄.
Larger variants of more recent models like Parti are also better at producing natural text—it seems this is another emergent ability of large multi-modal models.
If you are envious of the high-quality generation of these closed large models, fear not. DALL-E mini is an open-source alternative created by Boris Dayma that reproduces DALL-E with a smaller architecture.
The model has become very popular lately with many websites covering how to get around high traffic errors. My favourite part about DALL-E mini are a reddit page and Twitter account that chronicle the often hilariously weird and surreal creations of the model.